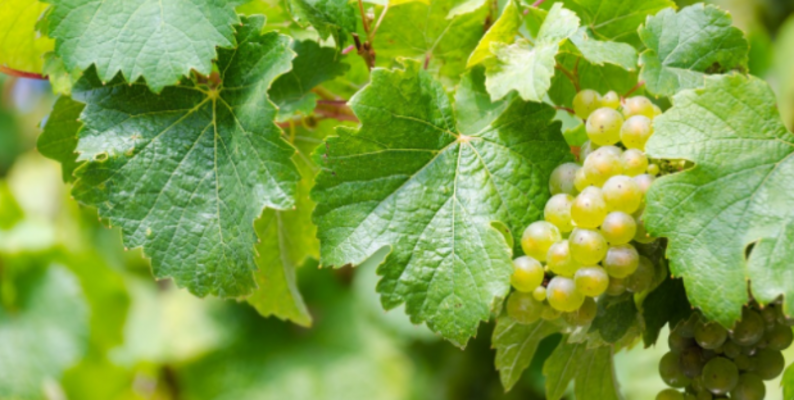
500 Instances
Image Attributes
5 Class
Uploaded: 2022
Grapevine Leaves Image Dataset
Ak, Ala Idris, Büzgülü, Dimnit and Nazli Grapevine Leaves Image
Read More
653 Downloads
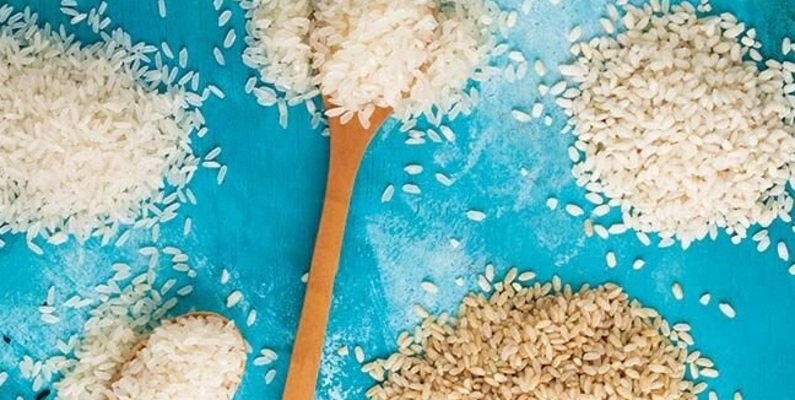
75000 Instances
106 Attributes
5 Class
Uploaded: 2021
Rice MSC Dataset
Five different Rice MSC Dataset. Arborio, Basmati, Ipsala, Jasmine, Karacadag
Read More
123 Downloads
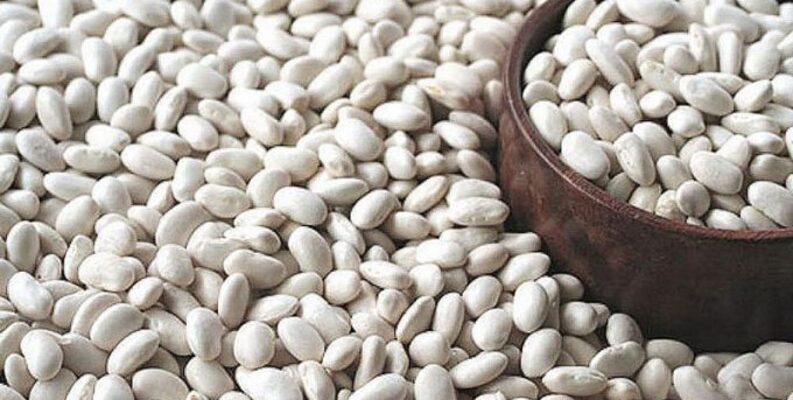
13644 Instances
16 Attributes
7 Class
Uploaded: 2020
Dry Bean Dataset
7 - Class: Seker, Barbunya, Bombay, Cali, Dermosan, Horoz and Sira
Read More
123 Downloads
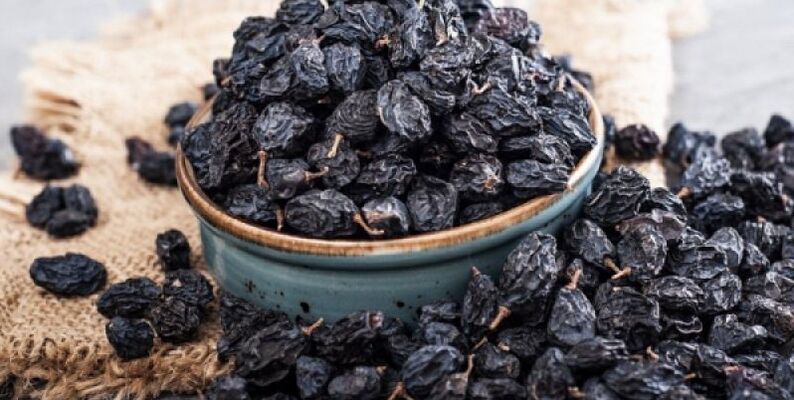
900 Instances
7 Attributes
2 Class
Uploaded: 2020
Raisin Dataset
Raisin Dataset; 2 Class: Kecimen and Besni Raisin
Read More
123 Downloads
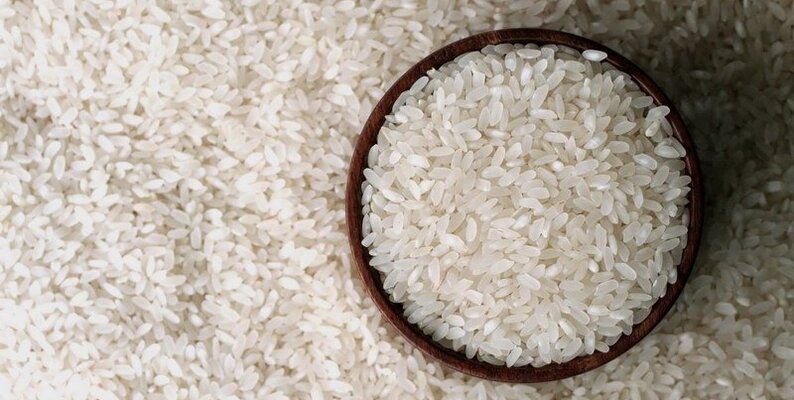
3810 Instances
9 Attributes
2 Class
Uploaded: 2019
Rice Dataset Cammeo and Osmancik
Rice Dataset: 2 Class Commeo and Osmancik Rice
Read More
231 Downloads