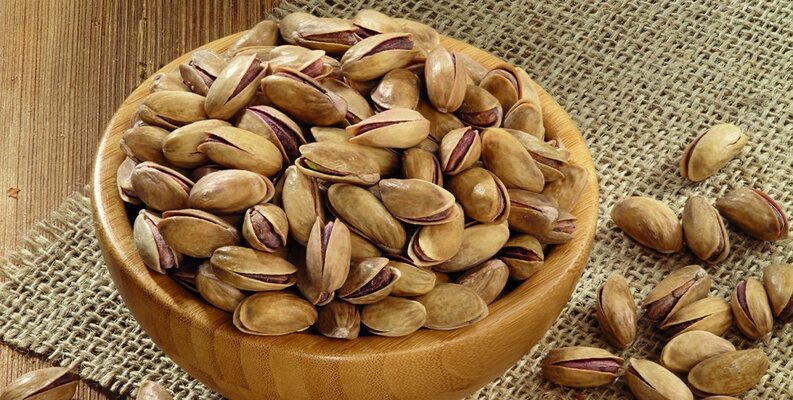
2148 Instances
Image Attributes
2 Class
Uploaded: 2022
Pistachio Image Dataset
The dataset includes a total of 2148 images, 1232 of Kirmizi and 916 of Siirt P.
Read More
100 Downloads
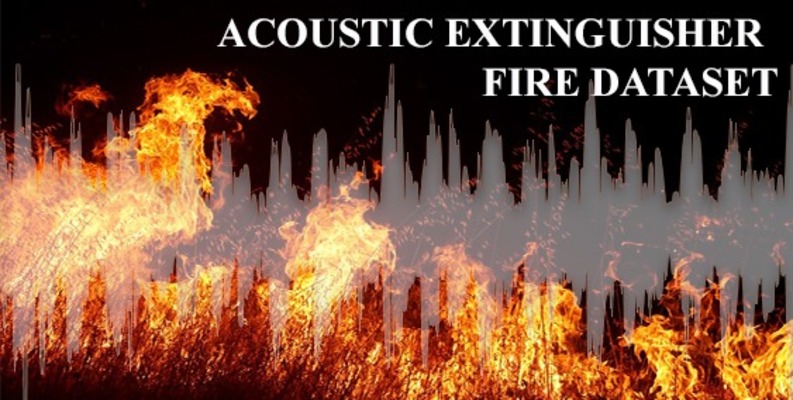
17442 Instances
6 Attributes
2 Class
Uploaded: 2022
Acoustic Extinguisher Fire Dataset
Acoustic Extinguisher Fire Dataset
Read More
159 Downloads
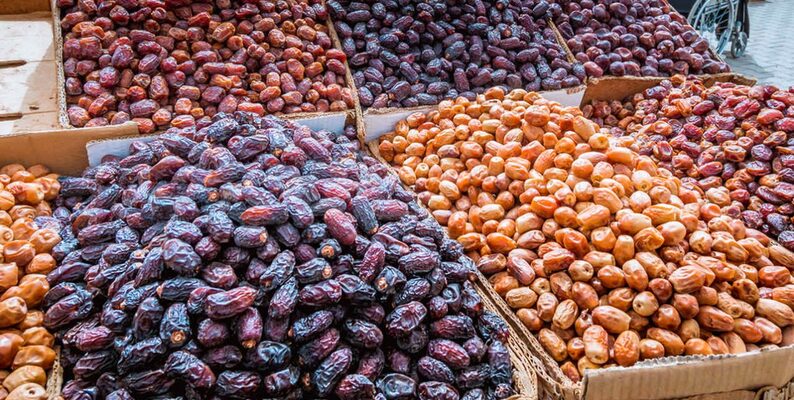
898 Instances
34 Attributes
7 Class
Uploaded: 2021
Date Fruit Datasets
7 Class; Barhee, Deglet Nour, Sukkary, Rotab Mozafati, Ruthana, Safawi, Sagai
Read More
2 Downloads
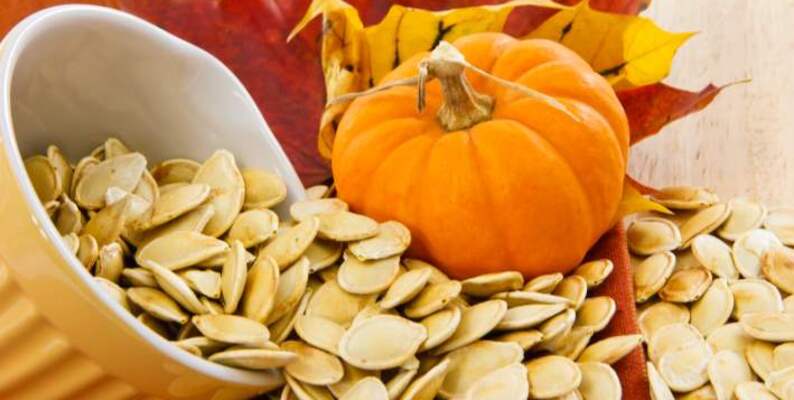
2500 Instances
12 Attributes
2 Class
Uploaded: 2021
Pumpkin Seeds Dataset
Pumpkin Seeds Dataset
Read More
650 Downloads
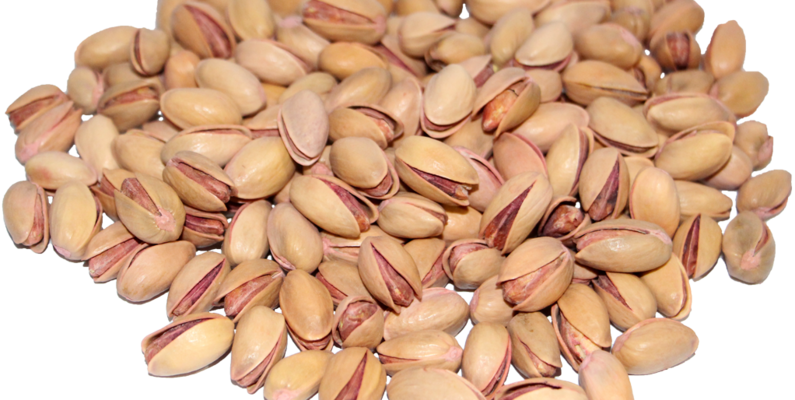
2148 Instances
16-28 Attributes
2 Class
Uploaded: 2021
Pistachio Dataset
Pistachio Dataset; 2 Class - Kirmizi and Siirt Pistachio, 16 and 28 Features
Read More
153 Downloads
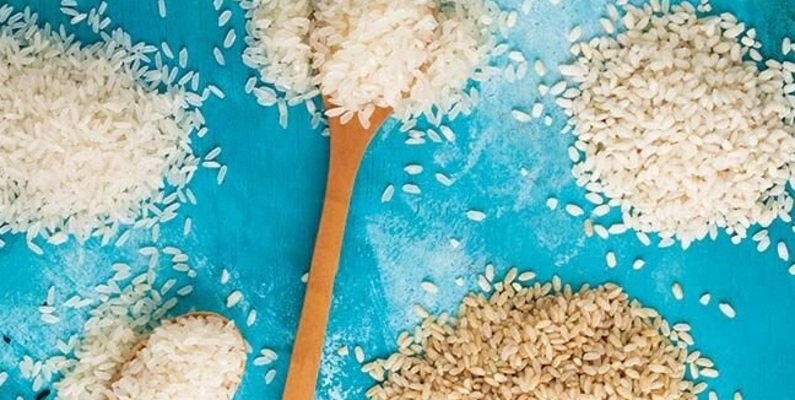
75000 Instances
106 Attributes
5 Class
Uploaded: 2021
Rice MSC Dataset
Five different Rice MSC Dataset. Arborio, Basmati, Ipsala, Jasmine, Karacadag
Read More
123 Downloads
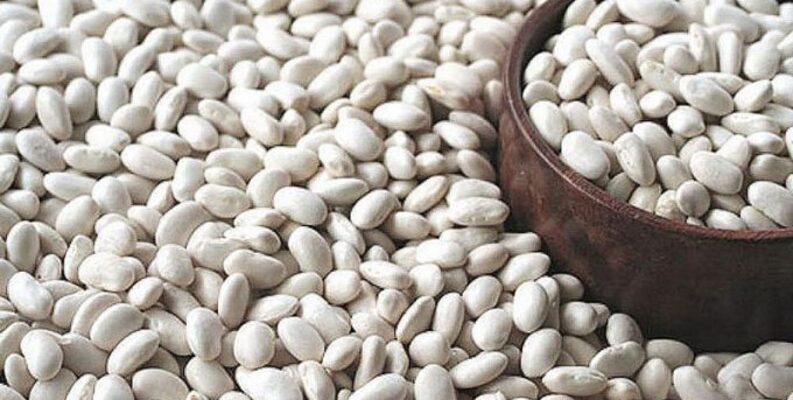
13644 Instances
16 Attributes
7 Class
Uploaded: 2020
Dry Bean Dataset
7 - Class: Seker, Barbunya, Bombay, Cali, Dermosan, Horoz and Sira
Read More
123 Downloads
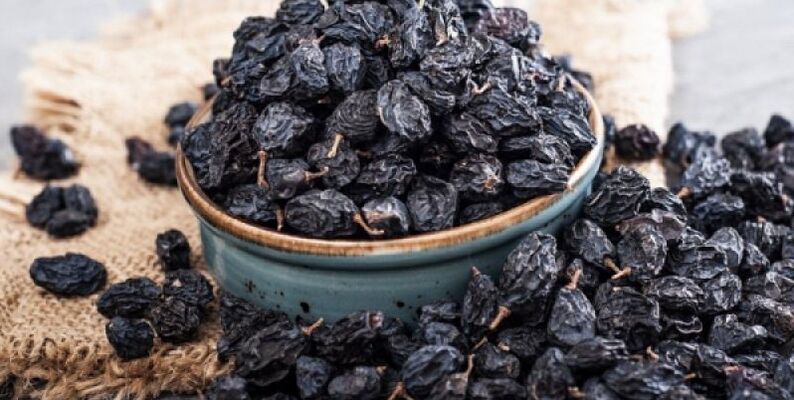
900 Instances
7 Attributes
2 Class
Uploaded: 2020
Raisin Dataset
Raisin Dataset; 2 Class: Kecimen and Besni Raisin
Read More
123 Downloads
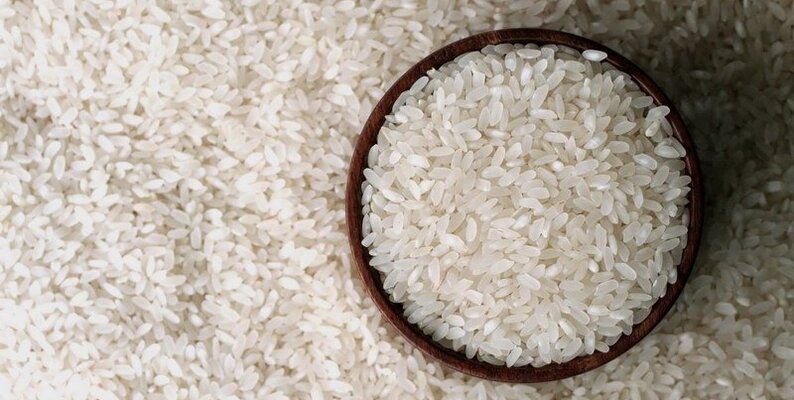
3810 Instances
9 Attributes
2 Class
Uploaded: 2019
Rice Dataset Cammeo and Osmancik
Rice Dataset: 2 Class Commeo and Osmancik Rice
Read More
231 Downloads
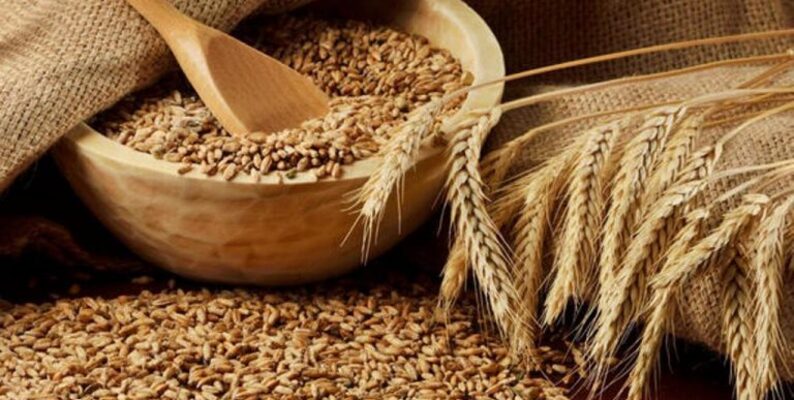
9000 Instances
236 Attributes
3 Class
Uploaded: 2019
Durum Wheat Dataset
Durum wheat dataset, morphological, colour, wavelet and gaborlet features
Read More
111 Downloads